How to perform dimensionality reduction on a mesh?#
Goal: get a lower-dimension representation of a mesh that can be fed to a regression model.
Hypotheses:
vertices are in one-to-one correspondence
Additional requirements:
pipeline should be invertible
pipeline must be compatible with
sklearn
[1]:
from pathlib import Path
import numpy as np
import pyvista as pv
from IPython.display import Image
from matplotlib import pyplot as plt
from sklearn.decomposition import PCA
from sklearn.preprocessing import FunctionTransformer, StandardScaler
from polpo.preprocessing import Map, NestingSwapper, PartiallyInitializedStep
from polpo.preprocessing.load.pregnancy import DenseMaternalMeshLoader
from polpo.preprocessing.mesh.io import PvReader
from polpo.preprocessing.mesh.registration import PvAlign
from polpo.sklearn.adapter import AdapterPipeline
from polpo.sklearn.mesh import InvertibleMeshesToVertices
from polpo.sklearn.np import InvertibleFlattenButFirst
[KeOps] Warning : cuda was detected, but driver API could not be initialized. Switching to cpu only.
[2]:
STATIC_VIZ = True
if STATIC_VIZ:
pv.set_jupyter_backend("static")
Loading meshes#
[3]:
prep_pipe = PartiallyInitializedStep(
Step=lambda **kwargs: Map(PvAlign(**kwargs)),
_target=lambda meshes: meshes[0],
max_iterations=500,
)
[4]:
subject_id = "01"
file_finder = DenseMaternalMeshLoader(
subject_id=subject_id,
as_dict=False,
left=True,
struct="Hipp",
)
pipe = file_finder + Map(PvReader()) + prep_pipe
meshes = pipe()
Create, fit and apply pipeline#
[5]:
pca = PCA(n_components=4)
objs2y = AdapterPipeline(
steps=[
InvertibleMeshesToVertices(index=0),
FunctionTransformer(func=np.stack),
InvertibleFlattenButFirst(),
StandardScaler(with_std=False),
pca,
],
)
objs2y
[5]:
AdapterPipeline(steps=[('step_0', InvertibleMeshesToVertices()), ('step_1', FunctionTransformer(func=<function stack at 0x75f868b9f1f0>)), ('step_2', InvertibleFlattenButFirst()), ('step_3', StandardScaler(with_std=False)), ('step_4', PCA(n_components=4))])In a Jupyter environment, please rerun this cell to show the HTML representation or trust the notebook.
On GitHub, the HTML representation is unable to render, please try loading this page with nbviewer.org.
AdapterPipeline(steps=[('step_0', InvertibleMeshesToVertices()), ('step_1', FunctionTransformer(func=<function stack at 0x75f868b9f1f0>)), ('step_2', InvertibleFlattenButFirst()), ('step_3', StandardScaler(with_std=False)), ('step_4', PCA(n_components=4))])
InvertibleMeshesToVertices()
FunctionTransformer(func=<function stack at 0x75f868b9f1f0>)
InvertibleFlattenButFirst()
StandardScaler(with_std=False)
PCA(n_components=4)
[6]:
objs2y.fit(meshes);
Let’s look at the explained variance ratio.
[7]:
plt.plot(np.cumsum(pca.explained_variance_ratio_), marker="o")
plt.ylabel("Cumulative Explained Variance Ratio");
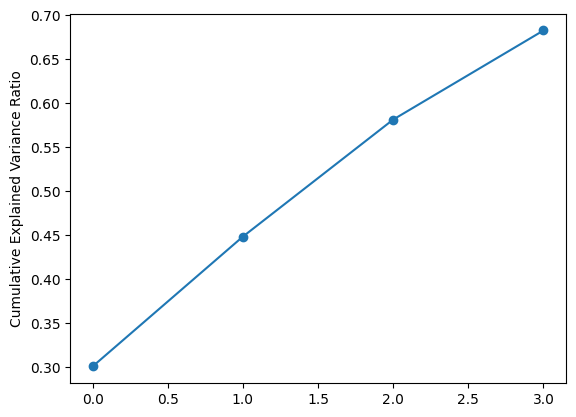
Visualize changes along the PCA axes#
This is how the hippocampus changes when we move along the PCA axes.
[8]:
comps = objs2y.transform(meshes)
mean_comps = comps.mean(axis=0)
rec_meshes = []
for comp_index in range(4):
sel_comps = comps[:, comp_index]
min_sel_comp, max_sel_comp = np.min(sel_comps), np.max(sel_comps)
var_comp = np.linspace(min_sel_comp, max_sel_comp, num=10)
X = np.broadcast_to(mean_comps, (len(var_comp), comps.shape[1])).copy()
X[:, comp_index] = var_comp
rec_meshes.append(objs2y.inverse_transform(X))
rec_meshes = NestingSwapper()(rec_meshes)
[10]:
outputs_dir = Path("_images")
if not outputs_dir.exists():
outputs_dir.mkdir()
gif_name = outputs_dir / "pca.gif"
pl = pv.Plotter(
shape=(2, 2),
border=False,
off_screen=True,
notebook=False,
)
pl.open_gif(gif_name.as_posix(), fps=3)
rendered_meshes = {}
for time_index, rec_meshes_ in enumerate(rec_meshes):
for comp_index, mesh in enumerate(rec_meshes_):
pl.subplot(comp_index // 2, comp_index % 2)
if time_index:
rendered_meshes[comp_index].points = mesh.points
else:
rendered_meshes[comp_index] = mesh_ = mesh.copy()
pl.add_mesh(mesh_, show_edges=True)
pl.add_title(f"{comp_index}", font_size=8)
pl.write_frame()
pl.close()
[11]:
Image(open(gif_name, "rb").read())
[11]:
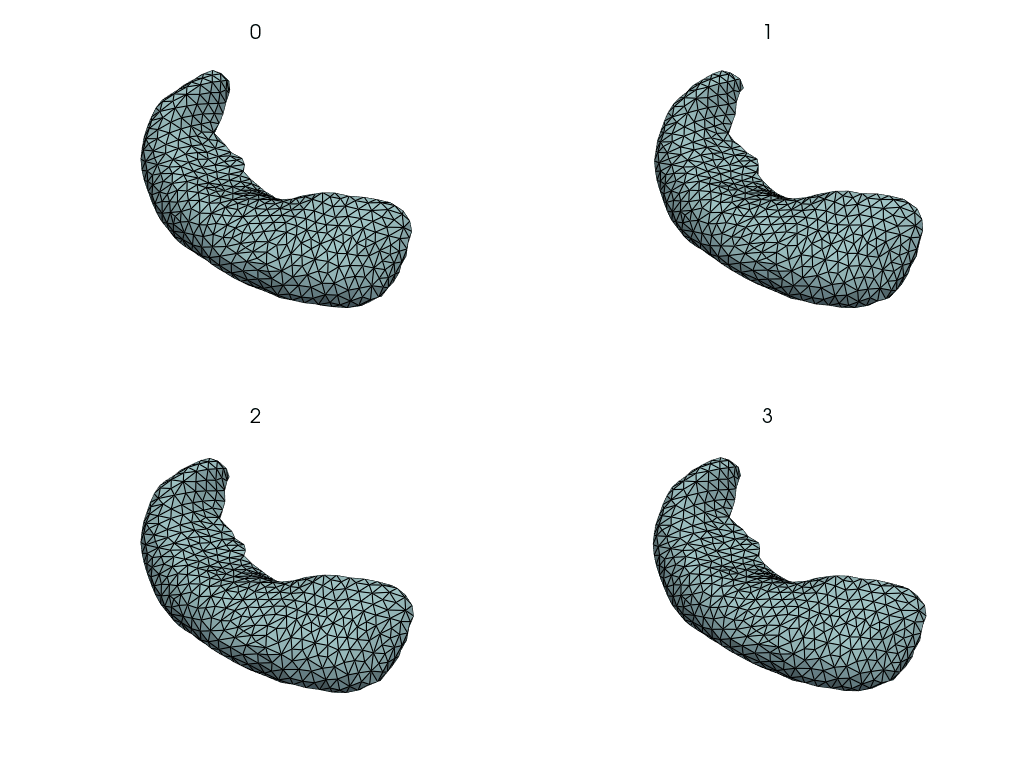